conf.researchr.org / Liyan Song
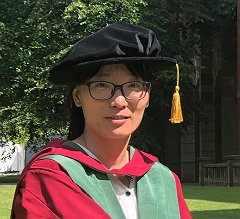
Registered user since Wed 2 Jun 2021
Name:Liyan Song
Bio:
Dr. Liyan Song is a Research Assistant Professor with the Southern University of Science and Technology, Shenzhen, China. Prior to that, she was a Research Fellow at the University of Birmingham, Birmingham, U.K. She received the B.Sc. and M.Sc. degrees in Mathematics from Harbin Institute of Technology, Harbin, China, and the Ph.D. degree in Computer Science from the University of Birmingham, Birmingham, UK. Her major research interests include data stream learning, class imbalance learning, unsupervised learning and their applications to predictive modelling in software engineering.
Country:China
Affiliation:Southern University of Science and Technology, China
Personal website: https://sunnysong14.github.io/
GitHub: https://github.com/sunnysong14
Research interests:software effort estimation, software defect prediction, machine learning
Contributions