LeGEND: A Top-Down Approach to Scenario Generation of Autonomous Driving Systems Assisted by Large Language Models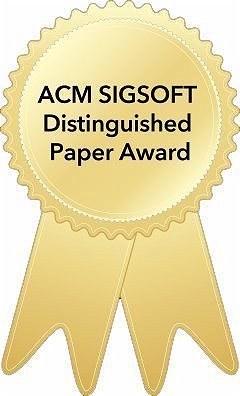
\emph{Autonomous driving systems (ADS)} are safety-critical and require comprehensive testing before their deployment on public roads. While existing testing approaches primarily focus on the criticality of scenarios, they often overlook the diversity of the generated scenarios that is also important to reflect system defects in different aspects. To bridge the gap, we propose Legend, that features a top-down fashion of scenario generation: it starts with abstract functional scenarios, and then step downwards to logical and concrete scenarios, such that scenario diversity can be controlled at the functional level.
However, unlike logical scenarios that can be formally described, functional scenarios are often documented in natural languages (e.g., accident reports) thus cannot be precisely parsed and processed by computers; to tackle that issue, Legend leverages the recent advances of large language models to transform functional scenarios in natural languages to formal logical scenarios. To mitigate the distraction of useless information in functional scenarios, we devise a two-stage transformation that features the use of an intermediate language; consequently, we adopt two LLMs in Legend, one for extracting information from functional scenarios, the other for converting the extracted information to formal logical scenarios. We experimentally evaluate Legend on Apollo, an industry-grade ADS from Baidu. Evaluation results show that Legend can effectively identify critical scenarios, and compared to baseline approaches, Legend exhibits evident superiority in diversity of generated scenarios. Moreover, we also demonstrate the advantages of our two-phase transformation framework, and the accuracy of the adopted LLMs.
Wed 30 OctDisplayed time zone: Pacific Time (US & Canada) change
13:30 - 15:00 | Autonomous SystemsResearch Papers / Journal-first Papers / Industry Showcase at Gardenia Chair(s): Qingkai Shi Nanjing University | ||
13:30 15mTalk | SoVAR: Build Generalizable Scenarios from Accident Reports for Autonomous Driving Testing Research Papers An Guo Nanjing University, Yuan Zhou Nanyang Technological University, Haoxiang Tian Nanyang Technological University, Chunrong Fang Nanjing University, Yunjian Sun Nanjing University, Weisong Sun Nanyang Technological University, Xinyu Gao , Luu Anh Tuan Nanyang Technological University, Yang Liu Nanyang Technological University, Zhenyu Chen Nanjing University Pre-print | ||
13:45 15mTalk | Bridging the Gap between Real-world and Synthetic Images for Testing Autonomous Driving Systems Research Papers | ||
14:00 15mTalk | In-Simulation Testing of Deep Learning Vision Models in Autonomous Robotic Manipulators Industry Showcase Dmytro Humeniuk Polytechnique Montréal, Houssem Ben Braiek Sycodal, Thomas Reid Sycodal, Foutse Khomh Polytechnique Montréal | ||
14:15 15mTalk | LeGEND: A Top-Down Approach to Scenario Generation of Autonomous Driving Systems Assisted by Large Language Models Research Papers Shuncheng Tang University of Science and Technology of China, Zhenya Zhang Kyushu University, Japan, Jixiang Zhou University of Science and Technology of China, Lei Wang National University of Defense Technology, Yuan Zhou Zhejiang Sci-Tech University, Yinxing Xue University of Science and Technology of China | ||
14:30 15mTalk | ROCAS: Root Cause Analysis of Autonomous Driving Accidents via Cyber-Physical Co-mutation Research Papers Shiwei Feng Purdue University, Yapeng Ye Purdue University, Qingkai Shi Nanjing University, Zhiyuan Cheng Purdue University, Xiangzhe Xu Purdue University, Siyuan Cheng Purdue University, Hongjun Choi DGIST, Xiangyu Zhang Purdue University | ||
14:45 15mTalk | The IDEA of Us: An Identity-Aware Architecture for Autonomous Systems Journal-first Papers Carlos Gavidia-Calderon The Alan Turing Institute, Anastasia Kordoni Lancaster University (UK), Amel Bennaceur The Open University, UK, Mark Levine Lancaster University, Bashar Nuseibeh The Open University, UK; Lero, University of Limerick, Ireland |