Phil Koopman
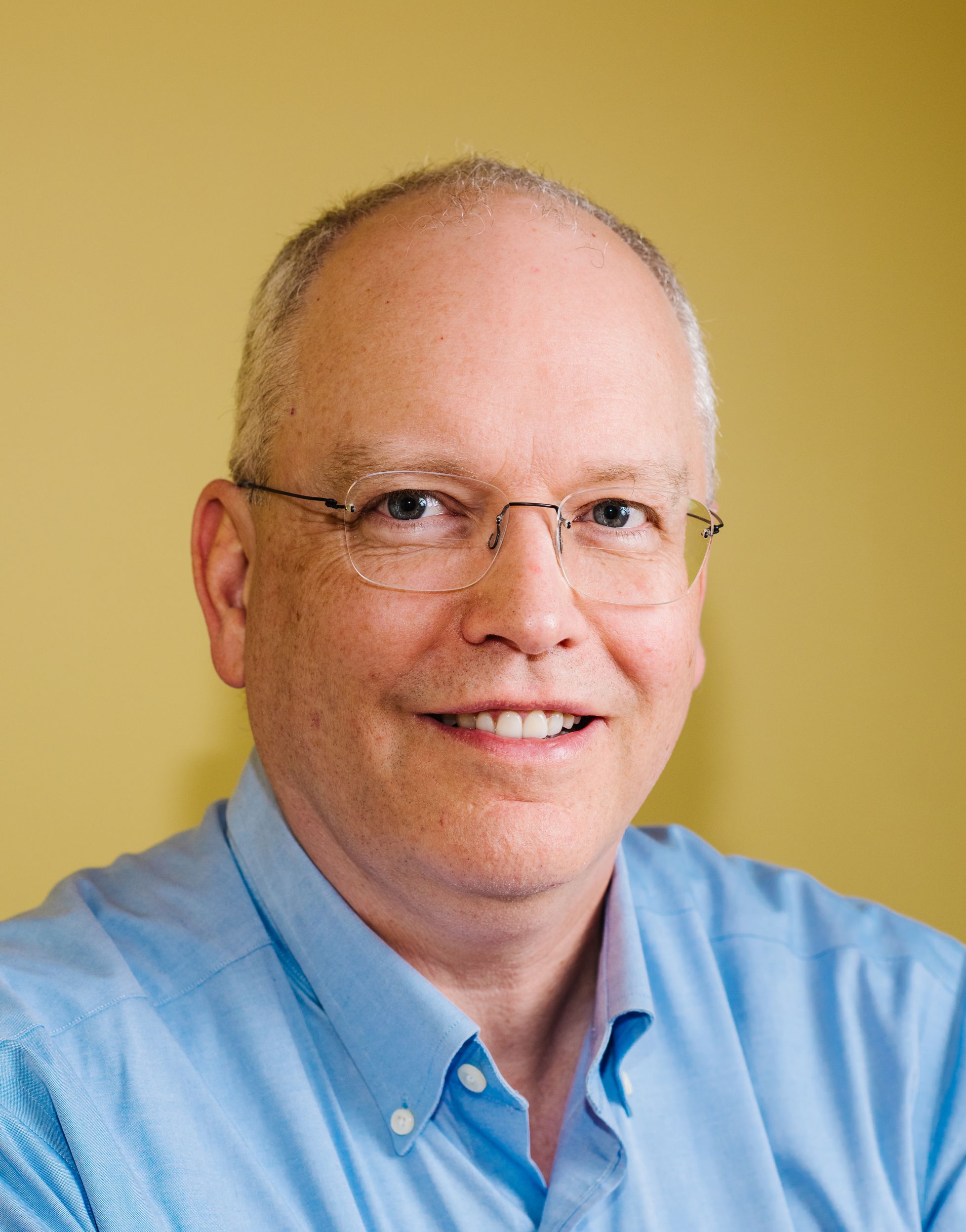
Safety Performance Indicators and Continuous Improvement Feedback
Abstract: Successful autonomous ground vehicles will require a continuous improvement strategy after deployment. Feedback from road testing and deployed operation will be required to ensure enduring safety in the face of newly discovered rare events. Additionally, the operational environment will change over time, requiring the system design to adapt to new conditions. The need for ensuring life critical safety is likely to limit the amount of real time adaptation that can be relied upon. Beyond runtime responses, lifecycle safety approaches will need to incorporate significant field engineering feedback based on safety performance indicator monitoring.
A continuous monitoring and improvement approach will require a fundamental shift in the safety world-view for automotive applications. Previously, a useful fiction was maintained that vehicles were safe for their entire lifecycle when deployed, and any safety defect was an unwelcome surprise. This approach too often provoked denial and minimization of the risk presented by evidence of operational safety issues so as to avoid expensive recalls and blame. In the future, the industry will need to embrace a model in which issues are proactively detected and corrected in a way that avoids most loss events, and that uses field incident data as a primary driver of improvement. Responding to automatically generated field incident reports to avoid later losses should be a daily practice in the normal course of business rather than evidence of an engineering mistake for which blame is assigned. This type of engineering feedback approach should complement any on-board runtime adaptation and fault mitigation.
Short Bio: Prof. Philip Koopman is an internationally recognized expert on Autonomous Vehicle (AV) safety whose work in that area spans over 25 years. He is also actively involved with AV policy and standards as well as more general embedded system design and software quality. His pioneering research work includes software robustness testing and run time monitoring of autonomous systems to identify how they break and how to fix them. He has extensive experience in software safety and software quality across numerous transportation, industrial, and defense application domains including conventional automotive software and hardware systems. He was the principal technical contributor to the UL 4600 standard for autonomous system safety issued in 2020. He is a faculty member of the Carnegie Mellon University ECE department where he teaches software skills for mission-critical systems. In 2018 he was awarded the highly selective IEEE-SSIT Carl Barus Award for outstanding service in the public interest for his work in promoting automotive computer-based system safety.
Ivana Dusparic
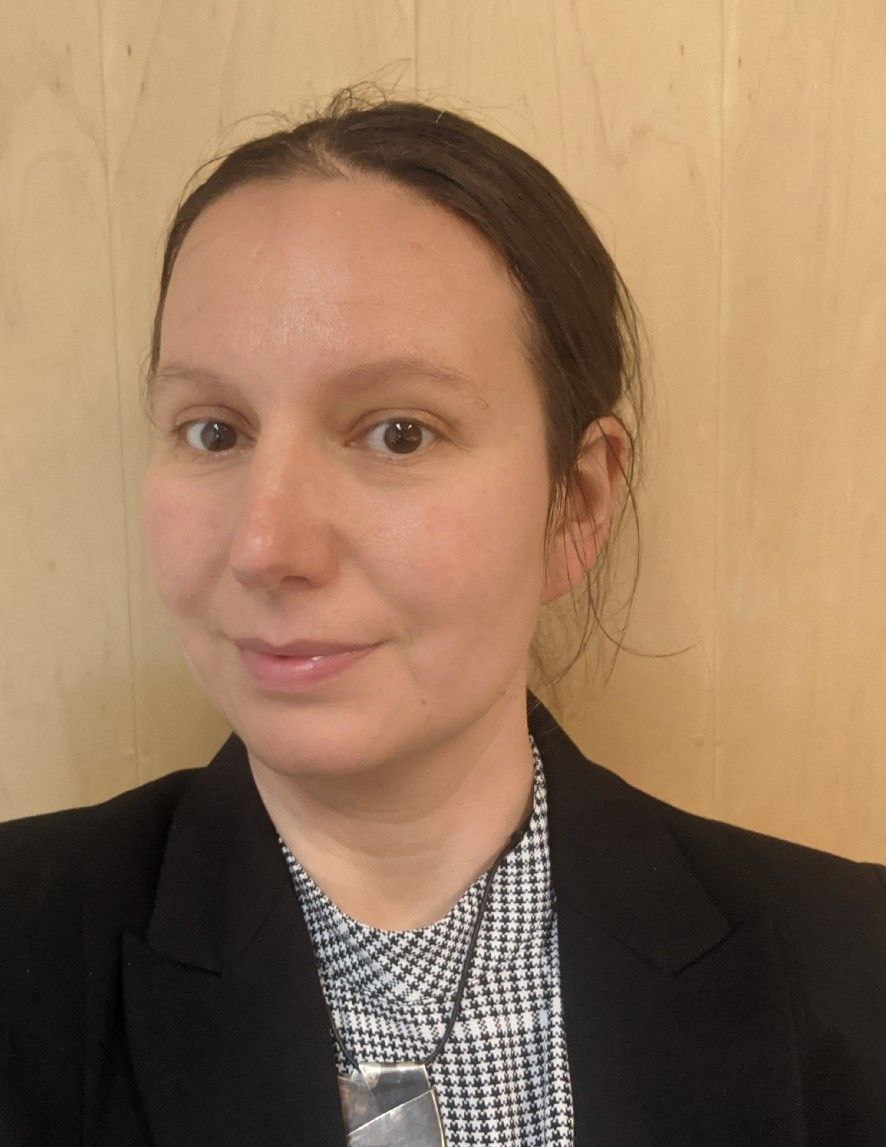
Reinforcement Learning for Self-Adaptation in Large-Scale Heterogeneous Dynamic Environments
Abstract: Reinforcement learning (RL), and in particular its combination with deep neural networks, has seen major breakthroughs in the recent years, most notably outperforming humans in games like Atari, Go, and StarCraft. RL use is also extensively investigated in a range of practical self-adaptive applications and cyber physical systems, however, existing algorithms often fall short of being suitable for use in such complex environments. My research focuses on developing techniques that enable the use of RL for optimization in large-scale adaptive systems, for example, urban traffic control, smart grid, and communication networks. These applications share properties with many other large-scale systems, i.e., are characterized by distributed control, heterogeneity, presence of multiple and often conflicting goals, reliance on diverse sources of information, and above all, the need for continuous adaptation. In this talk I will present a range of techniques for enabling the use of RL for optimization in such environments, in particular multi-agent multi-objective optimization, adaptation in non-stationary environments, online transfer learning, and state space adaptation. I will discuss further challenges in enabling RL deployment in self-adaptive systems, including development of new algorithms that can ensure seamless lifelong adaptivity and highlight the need for explainability and software testing techniques for RL-based applications.
Short bio: Reinforcement learning (RL), and in particular its combination with deep neural networks, has seen major breakthroughs in the recent years, most notably outperforming humans in games like Atari, Go, and StarCraft. RL use is also extensively investigated in a range of practical self-adaptive applications and cyber physical systems, however, existing algorithms often fall short of being suitable for use in such complex environments. My research focuses on developing techniques that enable the use of RL for optimization in large-scale adaptive systems, for example, urban traffic control, smart grid, and communication networks. These applications share properties with many other large-scale systems, i.e., are characterized by distributed control, heterogeneity, presence of multiple and often conflicting goals, reliance on diverse sources of information, and above all, the need for continuous adaptation. In this talk I will present a range of techniques for enabling the use of RL for optimization in such environments, in particular multi-agent multi-objective optimization, adaptation in non-stationary environments, online transfer learning, and state space adaptation. I will discuss further challenges in enabling RL deployment in self-adaptive systems, including development of new algorithms that can ensure seamless lifelong adaptivity and highlight the need for explainability and software testing techniques for RL-based applications.