conf.researchr.org / Xiaoning Du
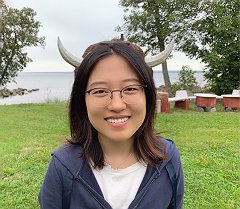
Registered user since Tue 18 Dec 2018
Name:Xiaoning Du
Bio:
Dr Xiaoning Du is currently a lecturer (a.k.a. Assistant Professor) under the Department of Software System and Cybersecurity, Faculty of Information Technology. She received her PhD from Nanyang Technological University in 2020 and her bachelor’s degree from Fudan University in 2014. Xiaoning specializes in software engineering, AI, and cybersecurity. Her research has bridged the gap between the theory and practical usage of program analysis and formal methods in the evaluation of traditional and AI-assisted software systems, aiming for better quality assurance and security. Her publications appear in top-tier venues including S&P, TDSC, ICSE, FSE, ASE, NeurIPS, AAAI, and FM.
Country:Australia
Affiliation:Monash University
Personal website: https://xiaoningdu.github.io/
X (Twitter): https://x.com/xiaoning_du
GitHub: https://github.com/xiaoningdu
Research interests:SE4AI, software analysis and testing
Contributions
2025
2024
APSEC
ISSTA
- Author of Testing Graph Database Systems with Graph-State Persistence Oracle within the Technical Papers-track
- Committee Member in Program Committee within the Technical Papers-track
- Session Chair of Code Transformation (part of Technical Papers)
- Author of FDI: Attack Neural Code Generation Systems through User Feedback Channel within the Technical Papers-track
- Author of AI Coders Are among Us: Rethinking Programming Language Grammar towards Efficient Code Generation within the Technical Papers-track
EnCyCriS
ICSE
International Conference on Program Comprehension
2023
ESEC/FSE
- Committee Member in Program Committee within the Artifacts-track
- Committee Member in Program Committee within the Research Papers-track
- Author of [Remote] CodeMark: Imperceptible Watermarking for Code Datasets against Neural Code Completion Models within the Research Papers-track
- Author of DistXplore: Distribution-guided Testing for Evaluating and Enhancing Deep Learning Systems within the Research Papers-track