Data-Driven Design and Evaluation of SMT Meta-Solving Strategies: Balancing Performance, Accuracy, and Cost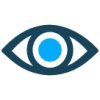
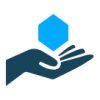
Many modern software engineering tools integrate SMT decision procedures and rely on the accuracy and performance of SMT solvers. We describe four basic patterns for integrating constraint solvers (earliest verdict, majority vote, feature-based solver selection, and verdict-based second attempt) that can be used for combining individual solvers into meta-decision procedures that balance accuracy, performance, and cost – or optimize for one of these metrics. In order to evaluate the effectiveness of meta-solving, we analyze and minimize 16 existing benchmark suites and benchmark seven state-of-the-art SMT solvers on 17k unique instances. From the obtained performance data, we can estimate the performance of different meta-solving strategies. We validate our results by implementing and analyzing one strategy. As additional results, we obtain (a) the first benchmark suite of unique SMT string problems with validated expected verdicts, (b) an extensive dataset containing data on benchmark instances as well as on the performance of individual decision procedures and several meta-solving strategies on these instances, and (c) a framework for generating data that can easily be used for similar analyses on different benchmark instances or for different decision procedures.