An Empirical Evaluation of Mutation Operators for Deep Learning Systems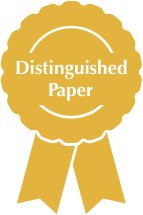
Sun 25 Oct 2020 22:00 - 22:30 at Farfetch (D. Maria) - RT3 - Testing Deep Learning and Robotic Systems Chair(s): João Pascoal Faria
Deep Learning (DL) is increasingly adopted to solve complex tasks such as image recognition or autonomous driving. Companies are considering the inclusion of DL components in production systems, but one of their main concerns is how to assess the quality of such systems. Mutation testing is a technique to inject artificial faults into a system, under the assumption that the capability to expose (kilt) such artificial faults translates into the capability to expose also real faults. Researchers have proposed approaches and tools (e.g., Deep-Mutation and MuNN) that make mutation testing applicable to deep learning systems. However, existing definitions of mutation killing, based on accuracy drop, do not take into account the stochastic nature of the training process (accuracy may drop even when re-training the un-mutated system). Moreover, the same mutation operator might be effective or might be trivial/impossible to kill, depending on its hyper-parameter configuration. We conducted an empirical evaluation of existing operators, showing that mutation killing requires a stochastic definition and identifying the subset of effective mutation operators together with the associated most effective configurations.
Sun 25 OctDisplayed time zone: Lisbon change
11:00 - 12:30 | RT3 - Testing Deep Learning and Robotic SystemsResearch Papers at Farfetch (D. Maria) +11h Chair(s): Antonio Filieri Imperial College London | ||
11:00 30mTalk | An Empirical Evaluation of Mutation Operators for Deep Learning Systems Research Papers Link to publication DOI | ||
11:30 30mTalk | Comparing Offline and Online Testing of Deep Neural Networks: An Autonomous Car Case Study Research Papers Fitash Ul Haq University of Luxembourg, Donghwan Shin University of Luxembourg, Shiva Nejati University of Luxembourg, Lionel Briand University of Luxembourg, University of Ottawa Link to publication DOI | ||
12:00 30mTalk | A Study on Challenges of Testing Robotic Systems Research Papers Afsoon Afzal Carnegie Mellon University, Claire Le Goues Carnegie Mellon University, Michael Hilton Carnegie Mellon University, USA, Christopher Steven Timperley Carnegie Mellon University Link to publication DOI |
22:00 - 23:30 | RT3 - Testing Deep Learning and Robotic SystemsResearch Papers at Farfetch (D. Maria) Chair(s): João Pascoal Faria Faculty of Engineering, University of Porto and INESC TEC | ||
22:00 30mTalk | An Empirical Evaluation of Mutation Operators for Deep Learning Systems Research Papers Link to publication DOI | ||
22:30 30mTalk | Comparing Offline and Online Testing of Deep Neural Networks: An Autonomous Car Case Study Research Papers Fitash Ul Haq University of Luxembourg, Donghwan Shin University of Luxembourg, Shiva Nejati University of Luxembourg, Lionel Briand University of Luxembourg, University of Ottawa Link to publication DOI | ||
23:00 30mTalk | A Study on Challenges of Testing Robotic Systems Research Papers Afsoon Afzal Carnegie Mellon University, Claire Le Goues Carnegie Mellon University, Michael Hilton Carnegie Mellon University, USA, Christopher Steven Timperley Carnegie Mellon University Link to publication DOI |