Codebase-Adaptive Detection of Security-Relevant Methods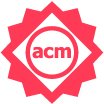
More and more companies use static analysis to perform regular code reviews to detect security vulnerabilities in their code, configuring them to detect various types of bugs and vulnerabilities such as the SANS top 25 or the OWASP top 10. For such analyses to be as precise as possible, they must be adapted to the code base they scan. The particular challenge we address in this paper is to provide analyses with the correct security-relevant methods (Srm): sources, sinks, etc. We present SWAN, a fully-automated machine-learning approach to detect sources, sinks, validators, and authentication methods for Java programs. SWAN further classifies the Srm into specific vulnerability classes of the SANS top 25. To further adapt the lists detected by SWAN to the code base and to improve its precision, we also introduce SWANAssist, an extension to SWAN that allows analysis users to refine the classifications. On twelve popular Java frameworks, SWAN achieves an average precision of 0.826, which is better or comparable to existing approaches. Our experiments show that SWANAssist requires a relatively low effort from the developer to significantly improve its precision.
Talk-Slides (20190718_SWAN.pdf) | 1.60MiB |
Thu 18 JulDisplayed time zone: Beijing, Chongqing, Hong Kong, Urumqi change
14:00 - 15:30 | Testing and Machine LearningTechnical Papers at Grand Ballroom Chair(s): Hongyu Zhang The University of Newcastle | ||
14:00 22mTalk | DeepHunter: A Coverage-Guided Fuzz Testing Framework for Deep Neural Networks Technical Papers Xiaofei Xie Nanyang Technological University, Lei Ma Kyushu University, Felix Juefei-Xu Carnegie Mellon University, Minhui Xue , Hongxu Chen Nanyang Technological University, Yang Liu Nanyang Technological University, Singapore, Jianjun Zhao Kyushu University, Bo Li UIUC, Jianxiong Yin NVIDIA AI Tech Centre, Simon See NVIDIA AI Tech Centre | ||
14:22 22mTalk | Search-based Test and Improvement of Machine-Learning-Based Anomaly Detection Systems Technical Papers Maxime Cordy SnT, University of Luxembourg, Steve Muller unaffiliated, Mike Papadakis University of Luxembourg, Yves Le Traon University of Luxembourg | ||
14:45 22mTalk | DeepFL: Integrating Multiple Fault Diagnosis Dimensions for Deep Fault Localization Technical Papers Xia Li University of Texas at Dallas, USA, Wei Li Southern University of Science and Technology, Yuqun Zhang Southern University of Science and Technology, Lingming Zhang | ||
15:07 22mTalk | Codebase-Adaptive Detection of Security-Relevant Methods Technical Papers Goran Piskachev Fraunhofer IEM, Lisa Nguyen Quang Do Paderborn University, Eric Bodden Heinz Nixdorf Institut, Paderborn University and Fraunhofer IEM DOI Pre-print Media Attached File Attached |